Failure prediction for multiple connected devices
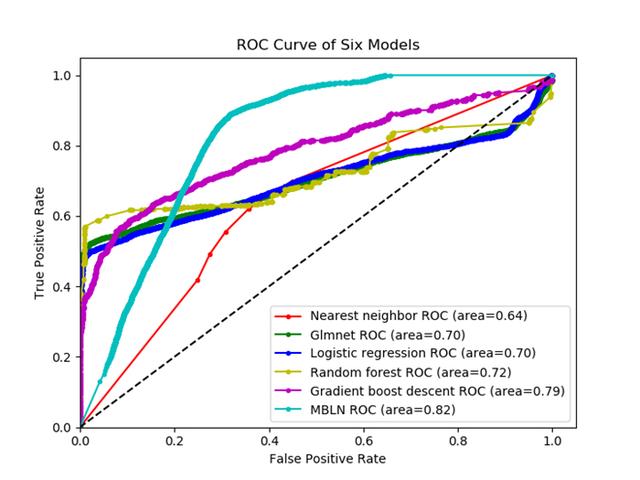
Failure prediction is an important problem in industry and has been studied over decades in various areas. Generally, majority of equipment and industrial components deteriorate after running for a period of time. The failures among multiple devices, which are physically connected to each other, may propagate. When a component of a system fails, other relevant components may break down too. For example, in a mill plant, when a motor fails, the bearings, which are physically connected to it, may fail as well. To capture these relationships, model-based techniques use system equations to extract analytical redundancies between devices’ measurements [1, 2]. An alternative approach is to apply a data-driven solution that uses information from similar devices for failure prediction. Data-driven methods use system’s measurements as the features for failure prediction [3]. In order to predict multivariate responses from multiple devices simultaneously with higher accuracy, we use the correlated features from these devices. We introduced multi-Bernoulli distribution with logit transformation to learn the correlation between the predictors and multivariate responses.