Explaining black box decisions by Shapley cohort refinement
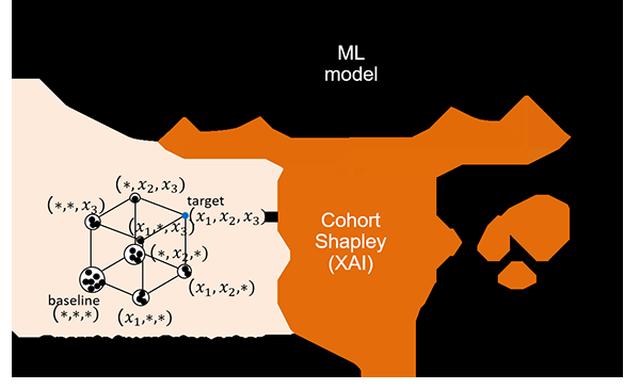
As AI prediction models continue to make increasingly accurate predictions, there is growing demand to apply them to mission critical tasks such as credit screening, crime prediction, fire risk assessment, and emergency medicine demand prediction. For such applications, recent complex prediction models are often criticized as black boxes as it is difficult to understand why the prediction model produces a given output. Explainable AI (XAI) has been intensively studied to resolve this dilemma, and ensure transparency. Post-hoc explanation methods such as LIME [1] and SHAP [2] has been proposed for explaining which input variables are important. The Shapley value [3] from cooperative game theory is gaining popularity for the explanation. It has a mathematically reasonable definition for globally consistent local explanation, and widely used, especially for tabular data. However, conventional Shapley based methods are potentially evaluating unlikely or even logically impossible synthetic data points where the machine learning prediction might be unreliable. Our cohort Shapley uses observed data points only. It realizes reliable explanations that matches human intuition.