Bayesian optimization algorithm with agent-based supply chain simulator for multi-echelon inventory management
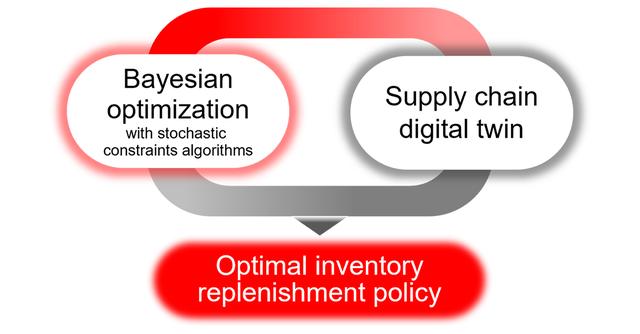
Having an efficient and resilient supply chain (SC) is critical for companies to stay competitive, and the disruptions caused by COVID-19 in the global supply chains have re-emphasized the importance of effective supply chain management (SCM). Among various SCM problems, inventory optimization that determines a replenishment policy (i.e., when and how much to order) with balanced inventory costs and service levels, is essential to ensure SC efficiency and increase customer satisfaction.
In practice, however, it is challenging to develop an optimized SC inventory policy. The reasons being, firstly, the SC structures are often complex since manufacturers with global businesses commonly use multiple tiers, or echelons, of stocking locations to minimize logistics costs, inventory costs, and sales opportunity loss. Next, apart from the complex multi-tier SC structure, other factors such as, the highly uncertain and rapidly changing market demand, the uncertain lead time between tiers, the tremendous variety of product types, and much-shortened product lifecycles, further complicate inventory optimization. Such practical factors make a conceptually simple inventory optimization problem, analytically intractable.
To analytically derive managerial insights, researchers have developed classical inventory management theories based on simplified assumptions such as static SC structure and stationary demand. These insights have been used in practice as the basis for a wide variety of heuristics but often result in sub-optimal SC performance because of violations of assumptions. To improve SC performance and solve analytically intractable real-world SC inventory optimization problems, researchers and practitioners have turned to simulation-based optimization approaches. Simulators, however, have traditionally been developed to solve a specific problem under consideration and are therefore not flexible or comprehensive enough to accommodate new dynamics such as SC structure changes mandated by the highly dynamic global market and increasing competition. Another challenge that hinders the practical usage of simulation-based optimization is that they are usually computationally expensive. This is because the deployed meta-heuristic optimization procedures such as the generic algorithm (GA) require many parameter evaluations when searching for the optimal SC parameters.
To enhance the applicability of the simulation-based optimization method in solving real-world SC inventory optimization problems, we developed Bayesian optimization approaches along with a flexible and comprehensive agent-based SC simulator. Numerical experiments have shown that our methods are much more efficient than popularly used GA.