Equipment Health Indicator Learning using Deep Reinforcement Learning
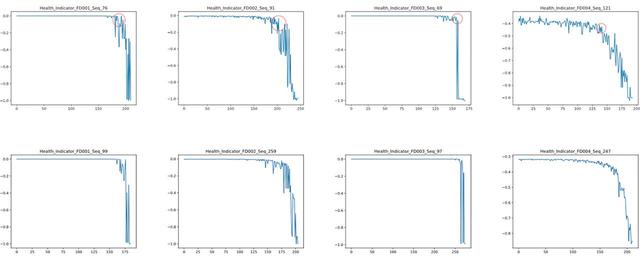
One of the important objectives in industrial operations is to minimize unexpected equipment failure. Unexpected downtime due to equipment failure can be very expensive for example, an unexpected failure of a mining truck in the field can have a cost of up to $5000 per hour on operations. Besides the cost, unexpected failures can cause safety and environmental hazards and lead to loss of life and property. Traditionally industries have tried to address this problem through time-based maintenance. However, time-based maintenance often causes over maintenance [1], while still not fully being able to address the problem of unplanned downtime. With the advent of IoT, Predictive Maintenance (PdM) is gaining popularity. One of the key applications of PdM implies predicting the future health status of equipment (e.g., failure prediction), and then taking proactive action based on the predictions.