Accelerating material properties determination with simulation-based machine learning
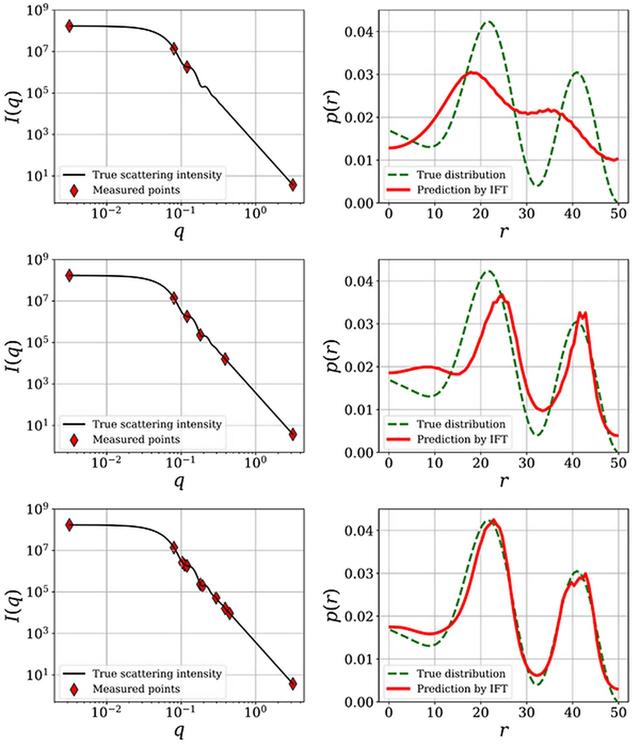
Innovation in materials has the potential to transform the society. For example, recyclable plastics could lead to a waste-free society, and advanced superconducting materials could enable new communication and cryptography systems. Investment in materials science and engineering is rising, notably as part of national initiatives in several countries. The Materials Genome Initiative [1] of the United States is a particularly famous example. In recent years, materials informatics (MI) has gained popularity as a data-driven approach to materials discovery and design [2]. While the success of MI hinges on materials datasets with wide coverage and high accuracy, such datasets are not yet sufficiently available in many fields of physics and chemistry. Thus, the demand for high-cost manual experiments to garner new data remains high. Precise determination of material properties often requires a sequence of elaborate measurements, and it is highly desired to expedite such experiments in order to reduce the time, money, and effort involved.