Generative adversarial networks for prognostics failure prediction
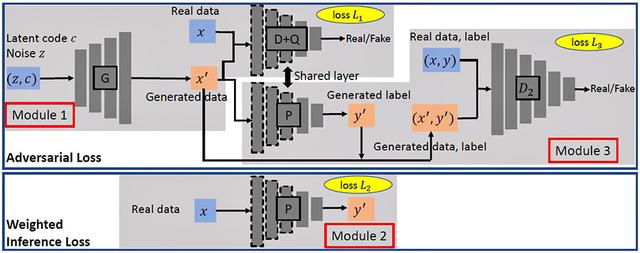
One of the main objectives of predictive maintenance is to predict and identify failures in equipment. This is typically done by monitoring the equipment and searching for any failure patterns. With the increased use of IoT devices, data-driven methods are increasingly being used to predict failures using large amount of sensor data collected from the system. To improve the accuracy of prediction however, requires sufficient samples of failure in the training data but represents a challenge as physical equipment and systems are engineered not to fail, thus failure data is rare and difficult to collect. In this blog, I’d like to talk about the Generative Adversarial Network (GAN) based approach to generate more samples to improve prediction result.