Wisdom of the ensemble: Improving consistency of deep learning models
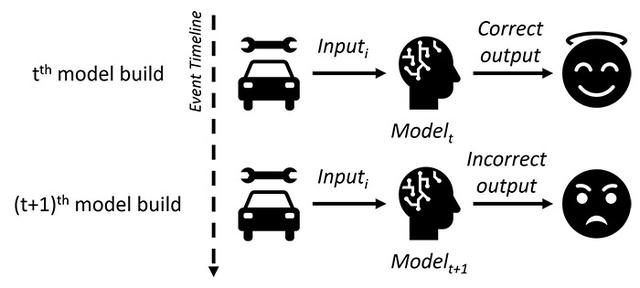
Building trustworthy AI systems is of paramount importance with its growing use [1]. One of the tenets to building trustworthy AI is the need for AI models to consistently produce correct outputs for the same input [2]. Based on observations in field we realized that as we periodically retrain AI models, there are no guarantees that different generations of the model will be consistently correct when presented with the same input.
For example, consider a scenario of model re-training, as shown in Figure 1, of a vehicle repair-recommendation system where given required inputs, the system returns a correct output – 'replace E.G.R valve.' A month later once the model is retrained, for the same input information, the system returns an incorrect output – ‘replace turbo speed sensor.’ This can lead to a faulty or even fatal repair, thus reducing the reliability and users’ trust in the underlying AI model. Similarly, consider the damage that can be caused by an AI-agent for COVID-19 diagnosis that correctly recommends a true patient to self-isolate, and subsequently changes its recommendation after being retrained with more data.