Making space for the data economy - A machine learning solution for data compression
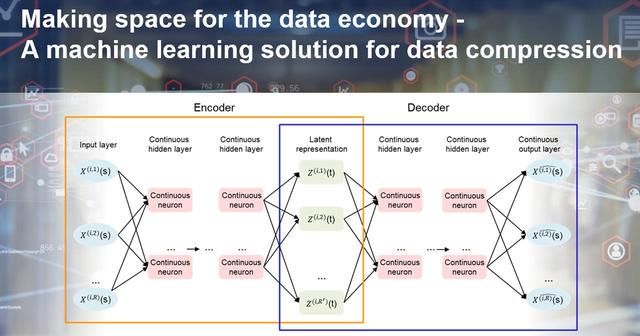
The global datasphere is growing at an unprecedented rate, with predictions that it will reach 175 zettabytes by 2025[1] but as a 2021 MIT Technology Review notes, we’re just “scratching the surface of data-driven opportunities.”[2] This presents exciting opportunities for data scientists, but it also raises challenges in terms of efficiently storing, transferring, and computing such vast amounts of data. Dimension reduction is a crucial technique in addressing these challenges, as it allows for the reduction of input variables while preserving meaningful data. In this blog article, we explore the limitations of existing dimension reduction methods and introduce a novel and effective approach using Functional Data Analysis (FDA) and Bi-Functional Autoencoders (BFAE).