Using unsupervised activity pattern encoding to recognize human working activities
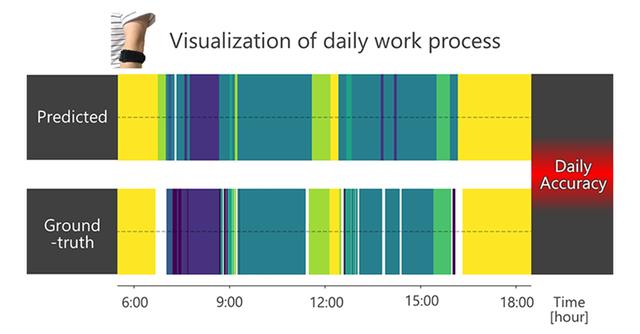
The widespread use of wearable sensors in recent years has enabled activity recognition (AR) to be studied in depth. Sensor data such as those from accelerometers is used in AR to process movement and recognize patterns, and has been successfully applied to the recognition of simple activities with characteristic motion, such as walking [1] or hand movement. As part of our goal to use AR to increase worker safety and security, and raise productivity and resilience, we are looking at how movement can be processed to recognize more complex activities. To recognize movement patterns in more complex activities such as working, we need AR of mid-working activities (e.g., eating assistance in caregivers) that will enable us to create and understand “worklogs” showing what is being done and how much time is being spent on that activity in a given work shift (Figure 1). However, despite the demand, working activity recognition (WAR) still faces numerous challenges particularly in the areas where big difference may occur between planned and actual works, such as in caregiving, construction, and so on.